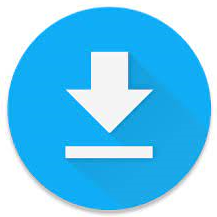

This section will share with you the 4 golden rules of creating a data visualization ppt your audience will understand.ĭata is only useful when your audience can understand it. That’s why visualizing data successfully is so important. Wouldn’t it be great to skip that overwhelmed feeling? Have you given that nervous smile and an unconvincing “Great, thanks”? Have you ever been handed a file full of data from a colleague and you have no idea what the data means? How to Visualize Data Like a Pro from 24Slides 4 Golden Rules of Creating a Data Visualization PowerPoint Presentation Read our 4 golden rules on how to make awesome visualizations or dive into our in depth guide in the topic. Popular types of data visualization include graphs, charts, and infographics. This type of information visualization allows people to add an extra dimension to their presentations and display important statistics in a cohesive manner.
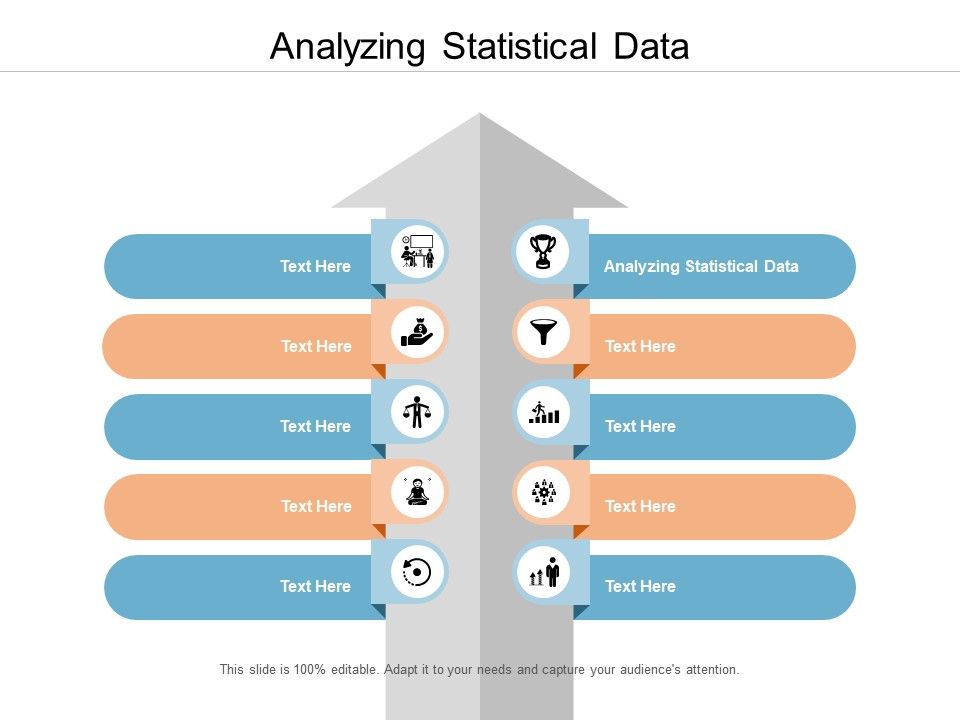
There are many different scenarios where large amounts of data must be displayed to an audience – a business may need to present sales figures to their directors, a research team may need to display their findings to investors, or a teacher may need to display statistics to their students for example.ĭata visualization allows this information to be displayed in an easy to read format that is both attractive and functional. Our theoretical results are verified by a series of numerical experiments including toy simulations and a real application of earthquake seismic intensity prediction.Data visualization is a pivotal part of a presentation.
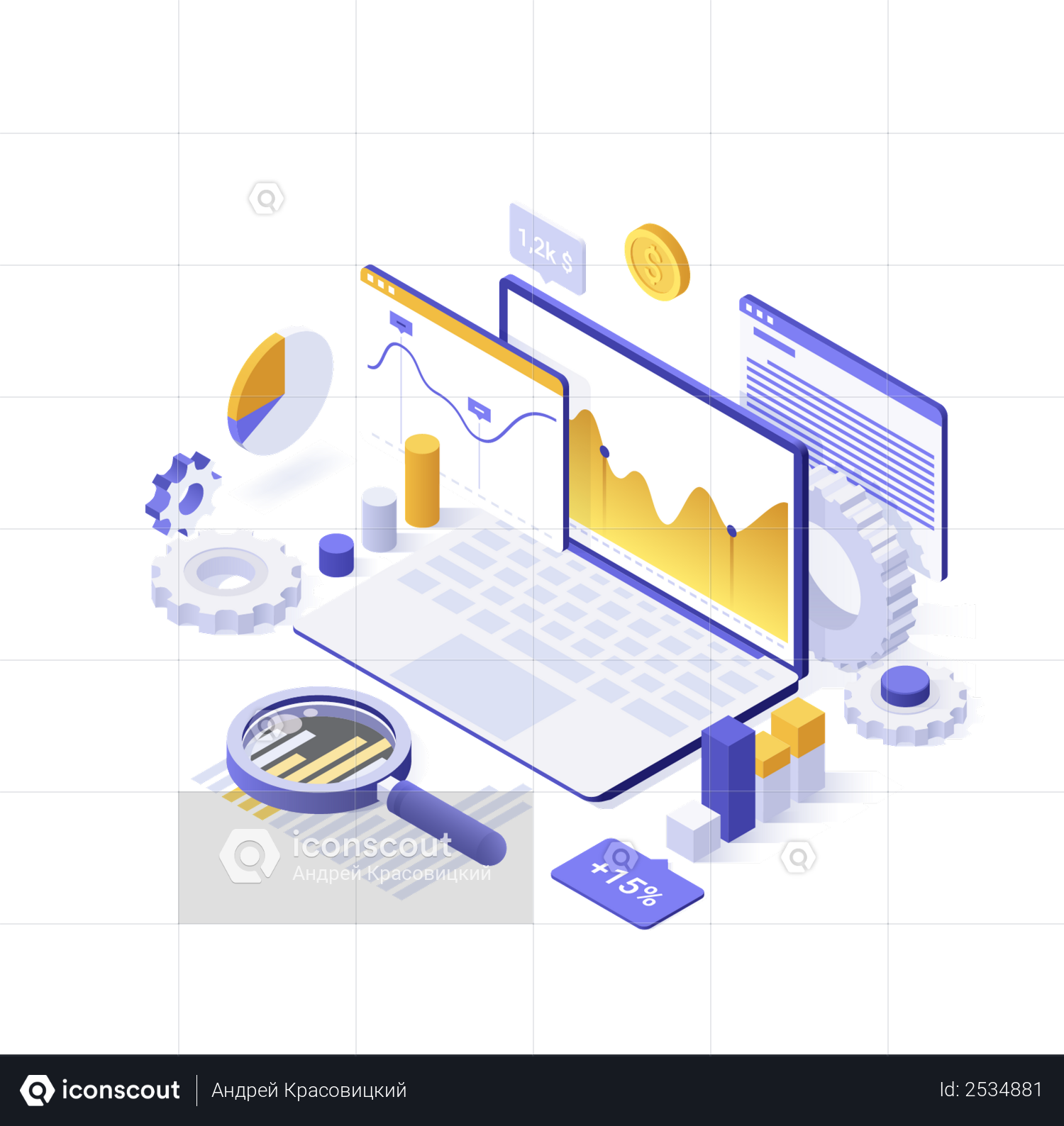
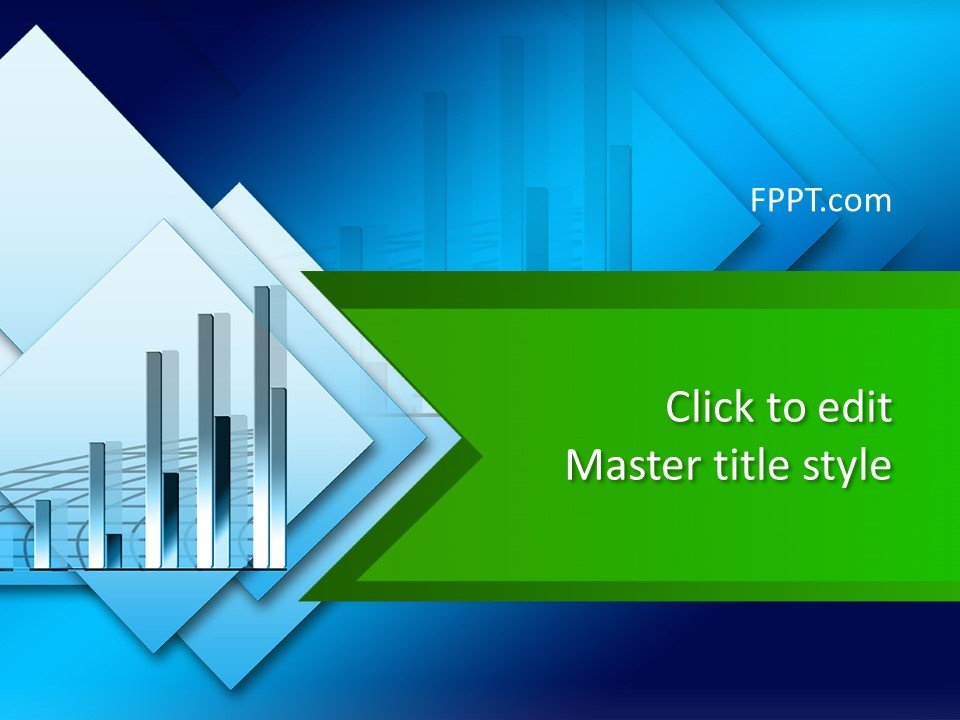
Based on these results, we prove that implementing the classical empirical risk minimization on deep nets can achieve the optimal generalization performance for numerous learning tasks. We present the adaptivity of features to depths and vice-verse via showing a depth-parameter trade-off in extracting both single feature and composite features. Our purpose is to quantify this feature-depth correspondence in feature extraction and generalization. One of the most important challenge of deep learning is to figure out relations between a feature and the depth of deep neural networks (deep nets for short) to reflect the necessity of depth. Thus it has triggered enormous research activities in machine learning and pattern recognition. Abstract : Deep learning is recognized to be capable of discovering deep features for representation learning and pattern recognition without requiring elegant feature engineering techniques by taking advantage of human ingenuity and prior knowledge.
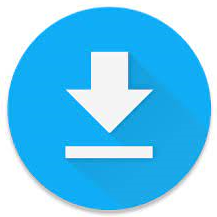